Science
How much more water and power does AI computing demand? Tech firms don't want you to know
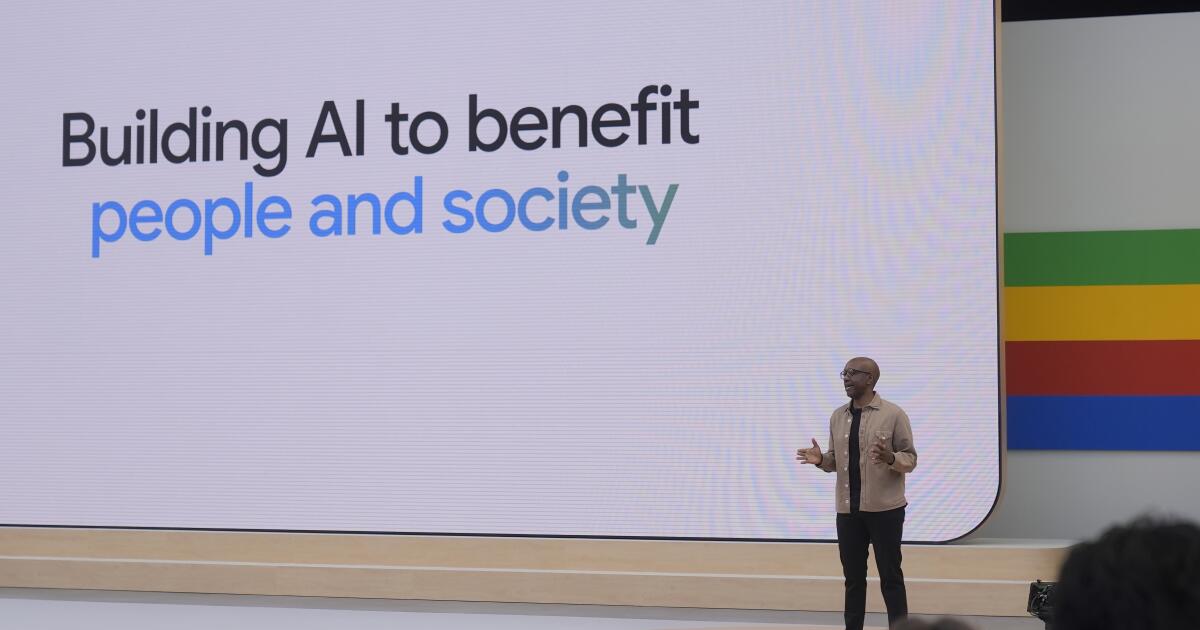
Every time someone uses ChatGPT to write an essay, create an image or advise them on planning their day, the environment pays a price.
A query on the chatbot that uses artificial intelligence is estimated to require at least 10 times more electricity than a standard search on Google.
If all Google searches similarly used generative AI, they might consume as much electricity as a country the size of Ireland, calculates Alex de Vries, the founder of Digiconomist, a website that aims to expose the unintended consequences of digital trends.
Yet someone using ChatGPT or another artificial intelligence application has no way of knowing how much power their questions will consume as they are processed in the tech companies’ enormous data centers.
De Vries said the skyrocketing energy demand of AI technologies will no doubt require the world to burn more climate-warming oil, gas and coal.
“Even if we manage to feed AI with renewables, we have to realize those are limited in supply, so we’ll be using more fossil fuels elsewhere,” he said. “The ultimate outcome of this is more carbon emissions.”
AI is also thirsty for water. ChatGPT gulps roughly a 16-ounce bottle in as few as 10 queries, calculates Shaolei Ren, associate professor of electrical and computer engineering at UC Riverside, and his colleagues.
The increasing consumption of energy and water by AI has raised concerns in California and around the globe. Experts have detailed how it could stall the transition to green energy, while increasing consumer’s electric bills and the risk of blackouts.
To try to prevent those consequences, De Vries, Ren and other experts are calling on the tech companies to disclose to users how much power and water their queries will consume.
“I think the first step is to have more transparency,” Ren said. The AI developers, he said, “tend to be secretive about their energy usage and their water consumption.”
Ren said users should be told on the websites where they are asked to type in their queries how much energy and water their requests will require. He said this would be similar to how Google now tells people searching for airline flights how much carbon emissions the trip will generate.
“If we had that knowledge,” he said, “then we could make more informed decisions.”
Data centers — enormous warehouses of computer servers that support the internet — have long been big power users. But the specialized computer chips required for generative AI use far more electricity because they are designed to read through vast amounts of data.
The new chips also generate so much heat that even more power and water is needed to keep them cool.
Even though the benefits and risks of AI aren’t yet fully known, companies are increasingly incorporating the technology into existing products.
In May, for example, Google announced that it was adding what it called “AI Overviews” to its search engine. Whenever someone now types a question into Google search, the company’s AI generates an answer from the search results, which is highlighted at the top.
Not all of Google’s AI-generated answers have been correct, including when it told a user to add Elmer’s glue to pizza sauce to keep cheese from sliding off the crust.
But searchers who don’t want those AI-generated answers or want to avoid the extra use of power and water can’t turn off the feature.
“Right now, the user doesn’t have the option to opt out,” Ren said.
Google did not respond to questions from The Times.
OpenAI, the company that created ChatGPT, responded with a prepared statement, but declined to answer specific questions, such as how much power and water the chatbot used.
“AI can be energy-intensive and that’s why we are constantly working to improve efficiencies,” OpenAI said. “We carefully consider the best use of our computing power and support our partners’ efforts to achieve their sustainability goals. We also believe that AI can play a key role in accelerating scientific progress in the discovery of climate solutions.”
Three years ago, Google vowed to reach “net-zero” — where its emissions of greenhouse gases would be equal to what it removed — by 2030.
The company isn’t making progress toward that goal. In 2023, its total carbon emissions increased by 13%, the company disclosed in a July report. Since 2019, its emissions are up 48%.
“As we further integrate AI into our products, reducing emissions may be challenging due to increasing energy demands from the greater intensity of AI compute,” the company said in the report.
Google added that it expects its emissions to continue to rise before dropping sometime in the future. It did not say when that may be.
The company also disclosed that its data centers consumed 6.1 billion gallons of water in 2023 — 17% more than the year before.
“We’re committed to developing AI responsibly by working to address its environmental footprint,” the report said.
De Vries said he was disappointed Google had not disclosed in the report how much AI was adding to its power needs. The company said in the report that such a “distinction between AI and other workloads” would “not be meaningful.”
By not separately reporting the power use of AI, he said, it is impossible to calculate just how much more electricity Google search was now using with the addition of AI Overviews.
“While capable of delivering the required info,” he said, “they are now withholding it.”

Science
How to protect yourself from the smoke caused by L.A. wildfires

You don’t have to live close to a wildfire to be affected by its smoke. With severe winds fanning the fires in and around Pacific Palisades, the Pasadena foothills and Simi Valley, huge swaths of the Southland are contending with dangerous air quality.
Wildfire smoke can irritate your eyes, nose, throat and lungs. The soot may contain all kinds of dangerous pollutants, including some that may cause cancer. The tiniest particles in smoke can travel deep into your lungs or even enter your bloodstream.
Conditions like these aren’t good for anyone, but they’re particularly bad for people in vulnerable groups, including children, those with asthma or other respiratory conditions, people with heart disease and those who are pregnant.
Here’s what you should know to keep yourself safe.
Stay indoors
Minimize your exposure to unhealthy air by staying inside and keeping your doors and windows shut.
If you have a central heating and air conditioning system, you can keep your indoor air clean by turning it on and keeping it running. Make sure the fresh-air intake is closed so that you’re not drawing in outdoor air.
Keep your pets inside
They shouldn’t breathe the unhealthy air either.
Check your air filters
Clean filters work better than dirty ones, and high-efficiency filters work better than regular ones. The California Air Resources Board and the South Coast Air Quality Management District recommend filters with a MERV rating of 13 or higher.
You might consider using portable high-efficiency air cleaner in a room where you spend the most time. The U.S. Environmental Protection Agency has information about them here, and CARB has a list of certified cleaning devices here.
Don’t pollute your indoor air
That means no burning candles or incense. If your power is out and you need to see in the dark, you’re much better off with a flashlight or headlamp.
If you’re cold, bundle up. This is not the time to start a cozy fire in the fireplace. Don’t use a gas stove or wood-fired appliances, since these will make your indoor air quality worse, not better, the AQMD says.
The CDC also advises against vacuuming, since it can stir up dust and release fine particles into the air.
Take care when cleaning up
You don’t want your skin to come into contact with wildfire ash. That means you should wear long sleeves, pants, gloves, socks and shoes. The AQMD even wants you to wear goggles.
If you’re sweeping up ash outdoors, get a hose and mist it with water first. That will keep it from flying up in the air as you move it around. Once the ash is wet, sweep it up gently with a broom or mop. Bag it up in a plastic bag and throw it away.
It’s a good idea to wash your vehicles and outdoor toys if they’re covered in ash. Try not to send ashy water into storm drains. Direct the dirty water into ground areas instead, the AQMD advises.
Those with lung or heart problems should avoid clean-up activities.
Discard spoiled food…
If you lost power for a significant length of time, the food in your refrigerator or freezer may be spoiled.
Food kept in a fridge should stay safe for up to four hours if you’ve kept the door closed. If you’ve been without power for longer than that, you’ll need to toss all perishable items, including meat, poultry, fish, eggs, milk and cut fruits and vegetables. Anything with “an unusual smell, color, or texture” should be thrown out as well, according to the U.S. Centers for Disease and Control Prevention.
Refrigerated medicines should be OK unless the power was out for more than a day. Check the label to make sure.
…even if it was in the freezer
Your freezer may be in better shape, especially if it’s well-stocked. Items in a full freezer may be safe for up to 48 hours if it’s been kept shut, and a half-full freezer may be OK for up to 24 hours. (The frozen items help keep each other cold, so the more the better.)
If items have remained below 40 degrees Fahrenheit (4 degrees Celsius) or you can still see ice crystals in them, they may be OK to use or refreeze, according to the federal government’s food safety website.
Ice cream and frozen yogurt should be thrown out if the power goes out for any amount of time. Meat, poultry, seafood, eggs, milk and most other dairy products need to go if they were exposed to temperatures above 40 degrees F for two hours or longer. The same goes for frozen meals, casseroles, soups, stews and cakes, pies and pastries with custard or cheese fillings.
Fruit and fruit juices that have started to thaw can be refrozen unless they’ve started to get moldy, slimy or smell like yeast. Vegetables and vegetable juices should be discarded if they’ve been above 40 degrees F for six hours or more, even if they look and smell fine.
Breakfast items like waffles and bagels can be refrozen, as can breads, rolls, muffins and other baked goods without custard fillings.
Consider alternative shelter
If you’ve done everything you can but your eyes are still watering, you can’t stop coughing, or you just don’t feel well, seek alternative shelter where the air quality is better.
Hold off on vigorous exercise
Doing anything that would cause you to breathe in more deeply is a bad idea right now.
Mask up outdoors
If you need to be outside for an extended time, be sure to wear a high-quality mask. A surgical mask or cloth mask won’t cut it — health authorities agree that you should reach for an N95 or P-100 respirator with a tight seal.
Are young children at greater risk of wildfire smoke?
Very young children are especially vulnerable to the effects of wildfire smoke because their lungs are still rapidly developing. And because they breathe much faster than adults, they are taking in more toxic particulate matter relative to their tiny bodies, which can trigger inflammation, coughing and wheezing.
Any kind of air pollution can be dangerous to young children, but wildfire smoke is about 10 times as toxic for children compared to air pollution from burning fossil fuels, said Dr. Lisa Patel, clinical associate professor of pediatrics at Stanford Children’s Health. Young children with preexisting respiratory problems like asthma are at even greater risk.
Patel advises parents to keep their young children indoors as much as possible, create a safe room in their home with an air purifier, and try to avoid using gas stoves to avoid polluting the indoor air.
Children over the age of 2 should also wear a well-fitting KN95 mask if they will be outdoors for a long period of time. Infants and toddlers younger than that don’t need to mask up because it can be a suffocation risk, Patel said.
What are the risks for pregnant people?
Pregnant people should also take extra precautions around wildfire smoke, which can cross the placenta and affect a developing fetus. Studies have found that exposure to wildfire smoke during pregnancy can increase the risk of premature birth and low birth weight. Researchers have also linked the toxic chemicals in smoke with maternal health complications including hypertension and preeclampsia.
What about other high-risk populations?
Certain chronic diseases including asthma, chronic obstructive pulmonary disease or other respiratory conditions can also make you particularly vulnerable to wildfire smoke. People with heart disease, diabetes and chronic kidney disease should take extra care to breathe clean air, the CDC says. The tiny particles in wildfire smoke can aggravate existing health problems, and may make heart attacks or strokes more likely, CARB warns.
Get ready for the next emergency
Living in Southern California means another wildfire is coming sooner or later. To prepare for the bad air, you can:
- Stock up on disposable respirators, like N95 or P-100s.
- Have clean filters ready for your A/C system and change them out when things get smoky.
- Know how to check the air quality where you live and work. The AQMD has an interactive map that’s updated hourly. Just type in an address and it will zoom in on the location. You can also sign up to get air quality alerts by email or on your smartphone.
- Know where your fire extinguisher is and keep it handy.
- If you have a heart or lung condition, keep at least five days’ worth of medication on hand.
Times staff writer Karen Garcia contributed to this report.
Science
Punk and Emo Fossils Are a Hot Topic in Paleontology

Mark Sutton, an Imperial College London paleontologist, is not a punk.
“I’m more of a folk and country person,” he said.
But when Dr. Sutton pieced together 3-D renderings of a tiny fossil mollusk, he was struck by the spikes that covered its wormlike body. “This is like a classic punk hairstyle, the way it’s sticking up,” he thought. He called the fossil “Punk.” Then he found a similar fossil with downward-tipped spines reminiscent of long, side-swept “emo” bangs. He nicknamed that specimen after the emotional alt-rock genre.
On Wednesday, Dr. Sutton and his colleagues published a paper in the journal Nature formally naming the creatures as the species Punk ferox and Emo vorticaudum. True to their names, these worm-mollusks are behind something of an upset (if not quite “anarchy in the U.K.”) over scientists’ understanding of the origins of one of the biggest groups of animals on Earth.
In terms of sheer number of species, mollusks are second only to arthropods (the group that contains insects, spiders and crustaceans). The better-known half of the mollusk family tree, conchiferans, contains animals like snails, clams and octopuses. “The other half is this weird and wacky group of spiny things,” Dr. Sutton said. Some animals in this branch, the aculiferans, resemble armored marine slugs, while others are “obscure, weird molluscan worms,” he said.
Punk and Emo, the forerunners of today’s worm-mollusks, lived on the dark seafloor amid gardens of sponges, nearly 200 million years before the first dinosaurs emerged on land. Today, their ancient seafloor is a fossil site at the border between England and Wales.
The site is littered with rounded rocky nodules that “look a bit like potatoes,” Dr. Sutton said. “And then you crack them open, and some of them have got these fossils inside. But the thing is, they don’t really look like much at first.”
While the nodules can preserve an entire animal’s body in 3-D, the cross-section that becomes visible when a nodule is cracked open can be difficult to interpret “because you’re not seeing the full anatomy,” Dr. Sutton said.
Paleontologists can use CT scans to see parts of fossils still hidden in rock, essentially taking thousands of X-rays of the fossil and then stitching those X-ray slices together into one digital 3-D image. But in these nodules, the fossilized creatures and the rock surrounding them are too similar in density to be easily differentiated by X-rays. Instead, Dr. Sutton essentially recreated this process of slicing and imaging by hand.
“We grind away a slice at a time, take a photo, repeat at 20-micron intervals or so, and basically destroy but digitize the fossil as we go,” Dr. Sutton said. At the end of the process, the original fossil nodule is “a sad-looking pile of dust,” but the thousands of images, when painstakingly digitally combined, provide a remarkable picture of the fossil animal.
Punk and Emo’s Hot Topic-worthy spikes set them apart from other fossils from the aculiferan branch of the mollusk family. “We don’t know much about aculiferans, and it’s unusual to find out we’ve suddenly got two,” Dr. Sutton said.
Stewart Edie, the curator of fossil bivalves at the Smithsonian National Museum of Natural History, said that Punk and Emo’s bizarre appearances shook up a long-held understanding of how mollusks evolved. Traditionally, scientists thought that the group of mollusks containing snails, clams and cephalopods “saw all of the evolutionary action,” said Dr. Edie, who was not involved with the new discovery. “And the other major group, the aculiferans, were considerably less adventurous.” But Punk and Emo “buck that trend,” he said.
The new alt-rock aculiferans reveal the hidden diversity of their group in the distant past and raise questions about why their descendants make up such a small part of the mollusk class today. “This is really giving us an almost unprecedented window into the sorts of things that were actually around when mollusks were getting going,” Dr. Sutton said. “It’s just this little weird, unexpected, really clear view of what was going on in the early history of one of the most important groups of animals.”
Science
FDA sets limits for lead in many baby foods as California disclosure law takes effect
The U.S. Food and Drug Administration this week set maximum levels for lead in baby foods such as jarred fruits and vegetables, yogurts and dry cereal, part of an effort to cut young kids’ exposure to the toxic metal that causes developmental and neurological problems.
The agency issued final guidance that it estimated could reduce lead exposure from processed baby foods by about 20% to 30%. The limits are voluntary, not mandatory, for food manufacturers, but they allow the FDA to take enforcement action if foods exceed the levels.
It’s part of the FDA’s ongoing effort to “reduce dietary exposure to contaminants, including lead, in foods to as low as possible over time, while maintaining access to nutritious foods,” the agency said in a statement.
Consumer advocates, who have long sought limits on lead in children’s foods, welcomed the guidance first proposed two years ago, but said it didn’t go far enough.
“FDA’s actions today are a step forward and will help protect children,” said Thomas Galligan, a scientist with the Center for Science in the Public Interest. “However, the agency took too long to act and ignored important public input that could have strengthened these standards.”
The new limits on lead for children younger than 2 don’t cover grain-based snacks such as puffs and teething biscuits, which some research has shown contain higher levels of lead. And they don’t limit other metals such as cadmium that have been detected in baby foods.
The FDA’s announcement comes just one week after a new California law took effect that requires baby food makers selling products in California to provide a QR code on their packaging to take consumers to monthly test results for the presence in their product of four heavy metals: lead, mercury, arsenic and cadmium.
The change, required under a law passed by the California Legislature in 2023, will affect consumers nationwide. Because companies are unlikely to create separate packaging for the California market, QR codes are likely to appear on products sold across the country, and consumers everywhere will be able to view the heavy metal concentrations.
Although companies are required to start printing new packaging and publishing test results of products manufactured beginning in January, it may take time for the products to hit grocery shelves.
The law was inspired by a 2021 congressional investigation that found dangerously high levels of heavy metals in packaged foods marketed for babies and toddlers. Baby foods and their ingredients had up to 91 times the arsenic level, up to 177 times the lead level, up to 69 times the cadmium level, and up to five times the mercury level that the U.S. allows to be present in bottled or drinking water, the investigation found.
There’s no safe level of lead exposure for children, according to the U.S. Centers for Disease Control and Prevention. The metal causes “well-documented health effects,” including brain and nervous system damage and slowed growth and development. However, lead occurs naturally in some foods and comes from pollutants in air, water and soil, which can make it impossible to eliminate entirely.
The FDA guidance sets a lead limit of 10 parts per billion for fruits, most vegetables, grain and meat mixtures, yogurts, custards and puddings and single-ingredient meats. It sets a limit of 20 parts per billion for single-ingredient root vegetables and for dry infant cereals. The guidance covers packaged processed foods sold in jars, pouches, tubs or boxes.
Jaclyn Bowen, executive director of the Clean Label Project, an organization that certifies baby foods as having low levels of toxic substances, said consumers can use the new FDA guidance in tandem with the new California law: The FDA, she said, has provided parents a “hard and fast number” to consider a benchmark when looking at the new monthly test results.
But Brian Ronholm, director of food policy for Consumer Reports, called the FDA limits “virtually meaningless because they’re based more on industry feasibility and not on what would best protect public health.” A product with a lead level of 10 parts per billion is “still too high for baby food. What we’ve heard from a lot of these manufacturers is they are testing well below that number.”
The new FDA guidance comes more than a year after lead-tainted pouches of apple cinnamon puree sickened more than 560 children in the U.S. between October 2023 and April 2024, according to the CDC.
The levels of lead detected in those products were more than 2,000 times higher than the FDA’s maximum. Officials stressed that the agency doesn’t need guidance to take action on foods that violate the law.
Aleccia writes for the Associated Press. Gold reports for The Times’ early childhood education initiative, focusing on the learning and development of California children from birth to age 5. For more information about the initiative and its philanthropic funders, go to latimes.com/earlyed.
-
Business1 week ago
These are the top 7 issues facing the struggling restaurant industry in 2025
-
Culture1 week ago
The 25 worst losses in college football history, including Baylor’s 2024 entry at Colorado
-
Sports1 week ago
The top out-of-contract players available as free transfers: Kimmich, De Bruyne, Van Dijk…
-
Politics6 days ago
New Orleans attacker had 'remote detonator' for explosives in French Quarter, Biden says
-
Politics6 days ago
Carter's judicial picks reshaped the federal bench across the country
-
Politics4 days ago
Who Are the Recipients of the Presidential Medal of Freedom?
-
Health3 days ago
Ozempic ‘microdosing’ is the new weight-loss trend: Should you try it?
-
World1 week ago
Ivory Coast says French troops to leave country after decades