Science
When A.I.’s Output Is a Threat to A.I. Itself

The internet is becoming awash in words and images generated by artificial intelligence.
Sam Altman, OpenAI’s chief executive, wrote in February that the company generated about 100 billion words per day — a million novels’ worth of text, every day, an unknown share of which finds its way onto the internet.
A.I.-generated text may show up as a restaurant review, a dating profile or a social media post. And it may show up as a news article, too: NewsGuard, a group that tracks online misinformation, recently identified over a thousand websites that churn out error-prone A.I.-generated news articles.
In reality, with no foolproof methods to detect this kind of content, much will simply remain undetected.
All this A.I.-generated information can make it harder for us to know what’s real. And it also poses a problem for A.I. companies. As they trawl the web for new data to train their next models on — an increasingly challenging task — they’re likely to ingest some of their own A.I.-generated content, creating an unintentional feedback loop in which what was once the output from one A.I. becomes the input for another.
In the long run, this cycle may pose a threat to A.I. itself. Research has shown that when generative A.I. is trained on a lot of its own output, it can get a lot worse.
Here’s a simple illustration of what happens when an A.I. system is trained on its own output, over and over again:
This is part of a data set of 60,000 handwritten digits.
When we trained an A.I. to mimic those digits, its output looked like this.
This new set was made by an A.I. trained on the previous A.I.-generated digits. What happens if this process continues? After 20 generations of training new A.I.s on their predecessors’ output, the digits blur and start to erode.
After 30 generations, they converge into a single shape.
While this is a simplified example, it illustrates a problem on the horizon.
Imagine a medical-advice chatbot that lists fewer diseases that match your symptoms, because it was trained on a narrower spectrum of medical knowledge generated by previous chatbots. Or an A.I. history tutor that ingests A.I.-generated propaganda and can no longer separate fact from fiction.
Just as a copy of a copy can drift away from the original, when generative A.I. is trained on its own content, its output can also drift away from reality, growing further apart from the original data that it was intended to imitate.
In a paper published last month in the journal Nature, a group of researchers in Britain and Canada showed how this process results in a narrower range of A.I. output over time — an early stage of what they called “model collapse.”
The eroding digits we just saw show this collapse. When untethered from human input, the A.I. output dropped in quality (the digits became blurry) and in diversity (they grew similar).
How an A.I. that draws digits “collapses” after being trained on its own output
If only some of the training data were A.I.-generated, the decline would be slower or more subtle. But it would still occur, researchers say, unless the synthetic data was complemented with a lot of new, real data.
Degenerative A.I.
In one example, the researchers trained a large language model on its own sentences over and over again, asking it to complete the same prompt after each round.
When they asked the A.I. to complete a sentence that started with “To cook a turkey for Thanksgiving, you…,” at first, it responded like this:
Even at the outset, the A.I. “hallucinates.” But when the researchers further trained it on its own sentences, it got a lot worse…
An example of text generated by an A.I. model. After two generations, it started simply printing long lists.
An example of text generated by an A.I. model after being trained on its own sentences for 2 generations.
And after four generations, it began to repeat phrases incoherently. An example of text generated by an A.I. model after being trained on its own sentences for 4 generations.
“The model becomes poisoned with its own projection of reality,” the researchers wrote of this phenomenon.
This problem isn’t just confined to text. Another team of researchers at Rice University studied what would happen when the kinds of A.I. that generate images are repeatedly trained on their own output — a problem that could already be occurring as A.I.-generated images flood the web.
They found that glitches and image artifacts started to build up in the A.I.’s output, eventually producing distorted images with wrinkled patterns and mangled fingers.
When A.I. image models are trained on their own output, they can produce distorted images, mangled fingers or strange patterns.
A.I.-generated images by Sina Alemohammad and others.
“You’re kind of drifting into parts of the space that are like a no-fly zone,” said Richard Baraniuk, a professor who led the research on A.I. image models.
The researchers found that the only way to stave off this problem was to ensure that the A.I. was also trained on a sufficient supply of new, real data.
While selfies are certainly not in short supply on the internet, there could be categories of images where A.I. output outnumbers genuine data, they said.
For example, A.I.-generated images in the style of van Gogh could outnumber actual photographs of van Gogh paintings in A.I.’s training data, and this may lead to errors and distortions down the road. (Early signs of this problem will be hard to detect because the leading A.I. models are closed to outside scrutiny, the researchers said.)
Why collapse happens
All of these problems arise because A.I.-generated data is often a poor substitute for the real thing.
This is sometimes easy to see, like when chatbots state absurd facts or when A.I.-generated hands have too many fingers.
But the differences that lead to model collapse aren’t necessarily obvious — and they can be difficult to detect.
When generative A.I. is “trained” on vast amounts of data, what’s really happening under the hood is that it is assembling a statistical distribution — a set of probabilities that predicts the next word in a sentence, or the pixels in a picture.
For example, when we trained an A.I. to imitate handwritten digits, its output could be arranged into a statistical distribution that looks like this:
Distribution of A.I.-generated data
Examples of
initial A.I. output:
The distribution shown here is simplified for clarity.
The peak of this bell-shaped curve represents the most probable A.I. output — in this case, the most typical A.I.-generated digits. The tail ends describe output that is less common.
Notice that when the model was trained on human data, it had a healthy spread of possible outputs, which you can see in the width of the curve above.
But after it was trained on its own output, this is what happened to the curve:
Distribution of A.I.-generated data when trained on its own output
It gets taller and narrower. As a result, the model becomes more and more likely to produce a smaller range of output, and the output can drift away from the original data.
Meanwhile, the tail ends of the curve — which contain the rare, unusual or surprising outcomes — fade away.
This is a telltale sign of model collapse: Rare data becomes even rarer.
If this process went unchecked, the curve would eventually become a spike:
Distribution of A.I.-generated data when trained on its own output
This was when all of the digits became identical, and the model completely collapsed.
Why it matters
This doesn’t mean generative A.I. will grind to a halt anytime soon.
The companies that make these tools are aware of these problems, and they will notice if their A.I. systems start to deteriorate in quality.
But it may slow things down. As existing sources of data dry up or become contaminated with A.I. “slop,” researchers say it makes it harder for newcomers to compete.
A.I.-generated words and images are already beginning to flood social media and the wider web. They’re even hiding in some of the data sets used to train A.I., the Rice researchers found.
“The web is becoming increasingly a dangerous place to look for your data,” said Sina Alemohammad, a graduate student at Rice who studied how A.I. contamination affects image models.
Big players will be affected, too. Computer scientists at N.Y.U. found that when there is a lot of A.I.-generated content in the training data, it takes more computing power to train A.I. — which translates into more energy and more money.
“Models won’t scale anymore as they should be scaling,” said Julia Kempe, the N.Y.U. professor who led this work.
The leading A.I. models already cost tens to hundreds of millions of dollars to train, and they consume staggering amounts of energy, so this can be a sizable problem.
‘A hidden danger’
Finally, there’s another threat posed by even the early stages of collapse: an erosion of diversity.
And it’s an outcome that could become more likely as companies try to avoid the glitches and “hallucinations” that often occur with A.I. data.
This is easiest to see when the data matches a form of diversity that we can visually recognize — people’s faces:
This set of A.I. faces was created by the same Rice researchers who produced the distorted faces above. This time, they tweaked the model to avoid visual glitches.
A grid of A.I.-generated faces showing variations in their poses, expressions, ages and races.
This is the output after they trained a new A.I. on the previous set of faces. At first glance, it may seem like the model changes worked: The glitches are gone.
After one generation of training on A.I. output, the A.I.-generated faces appear more similar.
After two generations …
After two generations of training on A.I. output, the A.I.-generated faces are less diverse than the original image.
After three generations …
After three generations of training on A.I. output, the A.I.-generated faces grow more similar.
After four generations, the faces all appeared to converge.
After four generations of training on A.I. output, the A.I.-generated faces appear almost identical.
This drop in diversity is “a hidden danger,” Mr. Alemohammad said. “You might just ignore it and then you don’t understand it until it’s too late.”
Just as with the digits, the changes are clearest when most of the data is A.I.-generated. With a more realistic mix of real and synthetic data, the decline would be more gradual.
But the problem is relevant to the real world, the researchers said, and will inevitably occur unless A.I. companies go out of their way to avoid their own output.
Related research shows that when A.I. language models are trained on their own words, their vocabulary shrinks and their sentences become less varied in their grammatical structure — a loss of “linguistic diversity.”
And studies have found that this process can amplify biases in the data and is more likely to erase data pertaining to minorities.
Ways out
Perhaps the biggest takeaway of this research is that high-quality, diverse data is valuable and hard for computers to emulate.
One solution, then, is for A.I. companies to pay for this data instead of scooping it up from the internet, ensuring both human origin and high quality.
OpenAI and Google have made deals with some publishers or websites to use their data to improve A.I. (The New York Times sued OpenAI and Microsoft last year, alleging copyright infringement. OpenAI and Microsoft say their use of the content is considered fair use under copyright law.)
Better ways to detect A.I. output would also help mitigate these problems.
Google and OpenAI are working on A.I. “watermarking” tools, which introduce hidden patterns that can be used to identify A.I.-generated images and text.
But watermarking text is challenging, researchers say, because these watermarks can’t always be reliably detected and can easily be subverted (they may not survive being translated into another language, for example).
A.I. slop is not the only reason that companies may need to be wary of synthetic data. Another problem is that there are only so many words on the internet.
Some experts estimate that the largest A.I. models have been trained on a few percent of the available pool of text on the internet. They project that these models may run out of public data to sustain their current pace of growth within a decade.
“These models are so enormous that the entire internet of images or conversations is somehow close to being not enough,” Professor Baraniuk said.
To meet their growing data needs, some companies are considering using today’s A.I. models to generate data to train tomorrow’s models. But researchers say this can lead to unintended consequences (such as the drop in quality or diversity that we saw above).
There are certain contexts where synthetic data can help A.I.s learn — for example, when output from a larger A.I. model is used to train a smaller one, or when the correct answer can be verified, like the solution to a math problem or the best strategies in games like chess or Go.
And new research suggests that when humans curate synthetic data (for example, by ranking A.I. answers and choosing the best one), it can alleviate some of the problems of collapse.
Companies are already spending a lot on curating data, Professor Kempe said, and she believes this will become even more important as they learn about the problems of synthetic data.
But for now, there’s no replacement for the real thing.
About the data
To produce the images of A.I.-generated digits, we followed a procedure outlined by researchers. We first trained a type of a neural network known as a variational autoencoder using a standard data set of 60,000 handwritten digits. We then trained a new neural network using only the A.I.-generated digits produced by the previous neural network, and repeated this process in a loop 30 times.
To create the statistical distributions of A.I. output, we used each generation’s neural network to create 10,000 drawings of digits. We then used the first neural network (the one that was trained on the original handwritten digits) to encode these drawings as a set of numbers, known as a “latent space” encoding. This allowed us to quantitatively compare the output of different generations of neural networks. For simplicity, we used the average value of this latent space encoding to generate the statistical distributions shown in the article.

Science
Political stress: Can you stay engaged without sacrificing your mental health?
It’s been two weeks since Donald Trump won the presidential election, but Stacey Lamirand’s brain hasn’t stopped churning.
“I still think about the election all the time,” said the 60-year-old Bay Area resident, who wanted a Kamala Harris victory so badly that she flew to Pennsylvania and knocked on voters’ doors in the final days of the campaign. “I honestly don’t know what to do about that.”
Neither do the psychologists and political scientists who have been tracking the country’s slide toward toxic levels of partisanship.
Fully 69% of U.S. adults found the presidential election a significant source of stress in their lives, the American Psychological Assn. said in its latest Stress in America report.
The distress was present across the political spectrum, with 80% of Republicans, 79% of Democrats and 73% of independents surveyed saying they were stressed about the country’s future.
That’s unhealthy for the body politic — and for voters themselves. Stress can cause muscle tension, headaches, sleep problems and loss of appetite. Chronic stress can inflict more serious damage to the immune system and make people more vulnerable to heart attacks, strokes, diabetes, infertility, clinical anxiety, depression and other ailments.
In most circumstances, the sound medical advice is to disengage from the source of stress, therapists said. But when stress is coming from politics, that prescription pits the health of the individual against the health of the nation.
“I’m worried about people totally withdrawing from politics because it’s unpleasant,” said Aaron Weinschenk, a political scientist at the University of Wisconsin–Green Bay who studies political behavior and elections. “We don’t want them to do that. But we also don’t want them to feel sick.”
Modern life is full of stressors of all kinds: paying bills, pleasing difficult bosses, getting along with frenemies, caring for children or aging parents (or both).
The stress that stems from politics isn’t fundamentally different from other kinds of stress. What’s unique about it is the way it encompasses and enhances other sources of stress, said Brett Ford, a social psychologist at the University of Toronto who studies the link between emotions and political engagement.
For instance, she said, elections have the potential to make everyday stressors like money and health concerns more difficult to manage as candidates debate policies that could raise the price of gas or cut off access to certain kinds of medical care.
Layered on top of that is the fact that political disagreements have morphed into moral conflicts that are perceived as pitting good against evil.
“When someone comes into power who is not on the same page as you morally, that can hit very deeply,” Ford said.
Partisanship and polarization have raised the stakes as well. Voters who feel a strong connection to a political party become more invested in its success. That can make a loss at the ballot box feel like a personal defeat, she said.
There’s also the fact that we have limited control over the outcome of an election. A patient with heart disease can improve their prognosis by taking medicine, changing their diet, getting more exercise or quitting smoking. But a person with political stress is largely at the mercy of others.
“Politics is many forms of stress all rolled into one,” Ford said.
Weinschenk observed this firsthand the day after the election.
“I could feel it when I went into my classroom,” said the professor, whose research has found that people with political anxiety aren’t necessarily anxious in general. “I have a student who’s transgender and a couple of students who are gay. Their emotional state was so closed down.”
That’s almost to be expected in a place like Wisconsin, whose swing-state status caused residents to be bombarded with political messages. The more campaign ads a person is exposed to, the greater the risk of being diagnosed with anxiety, depression or another psychological ailment, according to a 2022 study in the journal PLOS One.
Political messages seem designed to keep voters “emotionally on edge,” said Vaile Wright, a licensed psychologist in Villa Park, Ill., and a member of the APA’s Stress in America team.
“It encourages emotion to drive our decision-making behavior, as opposed to logic,” Wright said. “When we’re really emotionally stimulated, it makes it so much more challenging to have civil conversation. For politicians, I think that’s powerful, because emotions can be very easily manipulated.”
Making voters feel anxious is a tried-and-true way to grab their attention, said Christopher Ojeda, a political scientist at UC Merced who studies mental health and politics.
“Feelings of anxiety can be mobilizing, definitely,” he said. “That’s why politicians make fear appeals — they want people to get engaged.”
On the other hand, “feelings of depression are demobilizing and take you out of the political system,” said Ojeda, author of “The Sad Citizen: How Politics is Depressing and Why it Matters.”
“What [these feelings] can tell you is, ‘Things aren’t going the way I want them to. Maybe I need to step back,’” he said.
Genessa Krasnow has been seeing a lot of that since the election.
The Seattle entrepreneur, who also campaigned for Harris, said it grates on her to see people laughing in restaurants “as if nothing had happened.” At a recent book club meeting, her fellow group members were willing to let her vent about politics for five minutes, but they weren’t interested in discussing ways they could counteract the incoming president.
“They’re in a state of disengagement,” said Krasnow, who is 56. She, meanwhile, is looking for new ways to reach young voters.
“I am exhausted. I am so sad,” she said. “But I don’t believe that disengaging is the answer.”
That’s the fundamental trade-off, Ojeda said, and there’s no one-size-fits-all solution.
“Everyone has to make a decision about how much engagement they can tolerate without undermining their psychological well-being,” he said.
Lamirand took steps to protect her mental health by cutting social media ties with people whose values aren’t aligned with hers. But she will remain politically active and expects to volunteer for phone-banking duty soon.
“Doing something is the only thing that allows me to feel better,” Lamirand said. “It allows me to feel some level of control.”
Ideally, Ford said, people would not have to choose between being politically active and preserving their mental health. She is investigating ways to help people feel hopeful, inspired and compassionate about political challenges, since these emotions can motivate action without triggering stress and anxiety.
“We want to counteract this pattern where the more involved you are, the worse you are,” Ford said.
The benefits would be felt across the political spectrum. In the APA survey, similar shares of Democrats, Republicans and independents agreed with statements like, “It causes me stress that politicians aren’t talking about the things that are most important to me,” and, “The political climate has caused strain between my family members and me.”
“Both sides are very invested in this country, and that is a good thing,” Wright said. “Antipathy and hopelessness really doesn’t serve us in the long run.”
Science
Video: SpaceX Unable to Recover Booster Stage During Sixth Test Flight

President-elect Donald Trump joined Elon Musk in Texas and watched the launch from a nearby location on Tuesday. While the Starship’s giant booster stage was unable to repeat a “chopsticks” landing, the vehicle’s upper stage successfully splashed down in the Indian Ocean.
Science
Alameda County child believed to be latest case of bird flu; source unknown
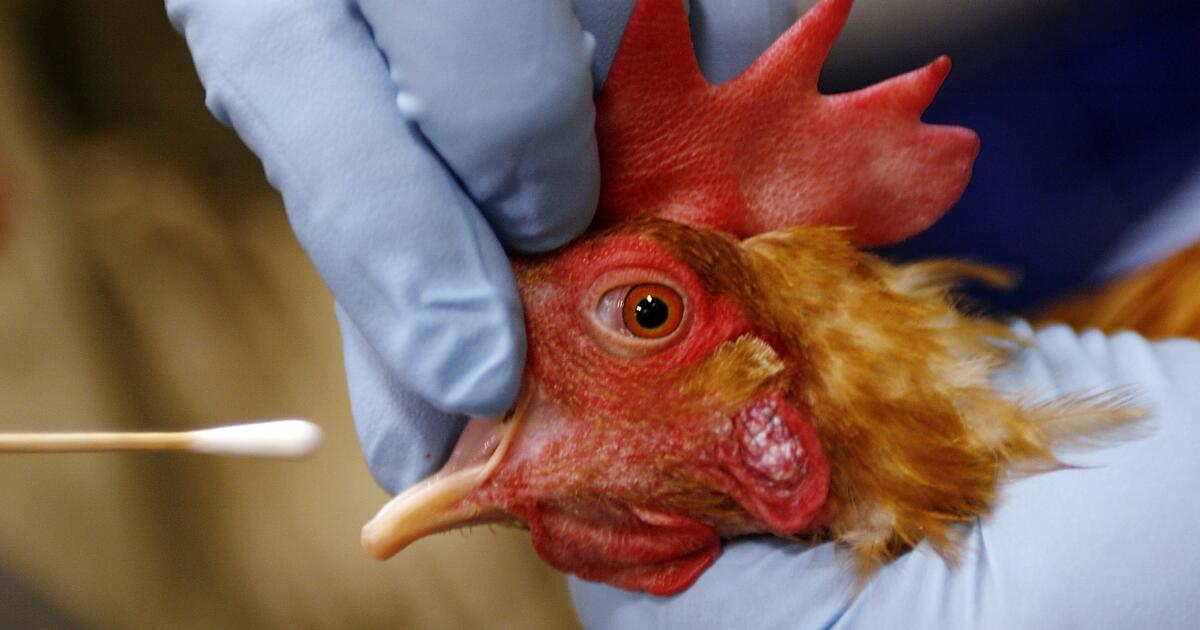
California health officials reported Tuesday that a child in Alameda County tested positive for H5 bird flu last week.
The source of infection is not known — although health officials are looking into possible contact with wild birds — and the child is recovering at home with mild upper respiratory symptoms.
Health officials have confirmed the “H5” part of the virus, not the “N1.” There is no human “H5” flu; it is only associated with birds.
The child was treated with antiviral medication, and the sample was sent to the U.S. Centers for Disease Control and Prevention for confirmatory testing.
The initial test showed low levels of the virus and, according to the state health agency, testing four days later showed no virus.
“The more cases we find that have no known exposure make it difficult to prevent additional” infections, said Jennifer Nuzzo, professor of epidemiology and director of the Brown University School of Public Health’s Pandemic Center. “It worries me greatly that this virus is popping up in more and more places and that we keep being surprised by infections in people whom we wouldn’t think would be at high risk of being exposed to the virus.”
A statement from the California Department of Public Health said that none of the child’s family members have the virus, although they, too, had mild respiratory symptoms. They are also being treated with antiviral medication.
The child attended a day care while displaying symptoms. People the child may have had contact with have been notified and are being offered preventative antiviral medication and testing.
“It’s natural for people to be concerned, and we want to reinforce for parents, caregivers and families that based on the information and data we have, we don’t think the child was infectious — and no human-to-human spread of bird flu has been documented in any country for more than 15 years,” said CDPH Director and State Public Health Officer Dr. Tomás Aragón.
The case comes days after the state health agency announced the discovery of six new bird flu cases, all in dairy workers. The total number of confirmed human cases in California is 27. This new case will bring it to 28, if confirmed. This is the first human case in California that is not associated with the dairy industry.
The total number of confirmed human cases in the U.S., including the Alameda County child, now stands at 54. Thirty-one are associated with dairy industry, 21 with the poultry industry, and now two with unknown sources.
In Canada, a teenager is in critical condition with the disease. The source of that child’s infection is also unknown.
Genetic sequencing of the Canadian teenager’s virus shows mutations that may make it more efficient at moving between people. The Canadian virus is also a variant of H5N1 that has been associated with migrating wild birds, not cattle.
Genetic sequencing of the California child’s virus has not been released, so it is unclear if it is of wild bird origin, or the one moving through the state’s dairy herds.
In addition, WastewaterScan — an infectious disease monitoring network led by researchers from Stanford University and Emory University, with laboratory support from Verily, Alphabet Inc.’s life sciences organization — follows 28 wastewater sites in California. All but six have shown detectable amounts of H5 in the last couple of weeks.
There are no monitoring sites in Alameda Co., but positive hits have been found in several Bay Area wastewater districts, including San Francisco, Redwood City, Sunnyvale, San Jose and Napa.
“This just makes the work of protecting people from this virus and preventing it from mutating to cause a pandemic that much harder,” said Nuzzo.
-
Business1 week ago
Column: OpenAI just scored a huge victory in a copyright case … or did it?
-
Health1 week ago
Bird flu leaves teen in critical condition after country's first reported case
-
Business5 days ago
Column: Molly White's message for journalists going freelance — be ready for the pitfalls
-
World1 week ago
Sarah Palin, NY Times Have Explored Settlement, as Judge Sets Defamation Retrial
-
Politics4 days ago
Trump taps FCC member Brendan Carr to lead agency: 'Warrior for Free Speech'
-
Science2 days ago
Trump nominates Dr. Oz to head Medicare and Medicaid and help take on 'illness industrial complex'
-
Technology3 days ago
Inside Elon Musk’s messy breakup with OpenAI
-
Lifestyle4 days ago
Some in the U.S. farm industry are alarmed by Trump's embrace of RFK Jr. and tariffs